*Yolov5 실험 결과 공유
*Model
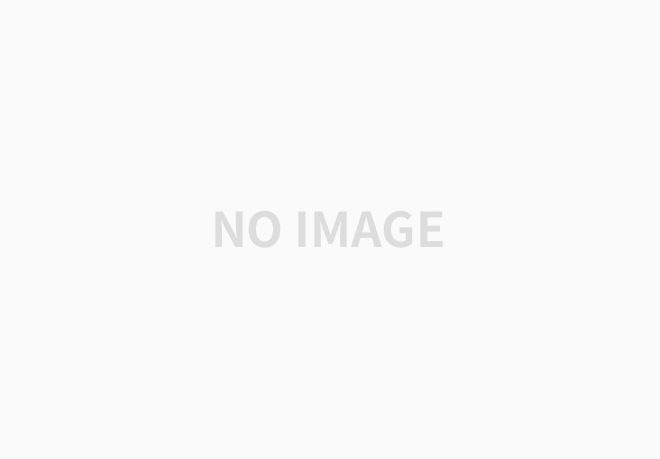
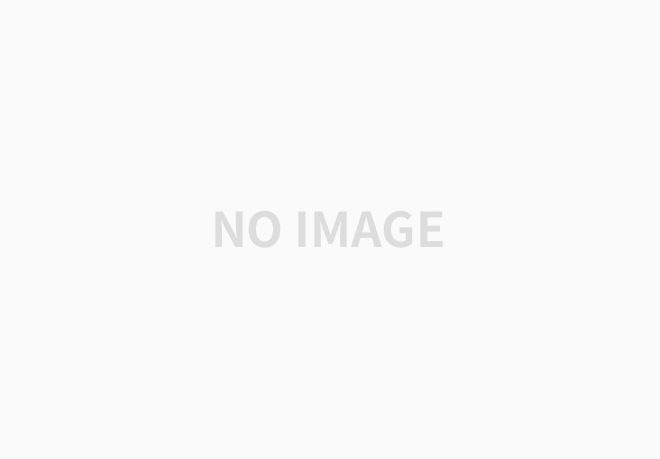
*Experiments
- configs (50epochs씩 총 4회 진행 : s , m , l , x )
- image size : 416 x 416 (default: 640 x 640)
- batch size : 32
- epochs : 50
- weights : yolov5s.pt (pretrained weights)
- optimizer : SGD
- scheduler : linear LR
- datasets : stratified train/valid
- augmentation : Default - Blur(p=0.01) , MedianBlur(p=0.01), ToGray(p=0.01), CLAHE (p=0.01)
*Results
- mAP
- yolo v5 s : 0.3938 (50epochs)
- yolo v5 m : 0.4189 (50epochs)
- yolo v5 l : 0.4438 (50epochs)
- yolo v5 x : 0.4668 (50epochs) -> submission하였음 (LB : 0.420)
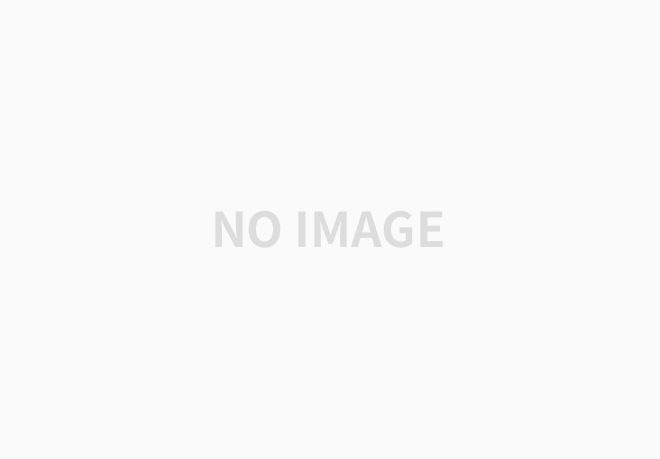
- Precision / Recall
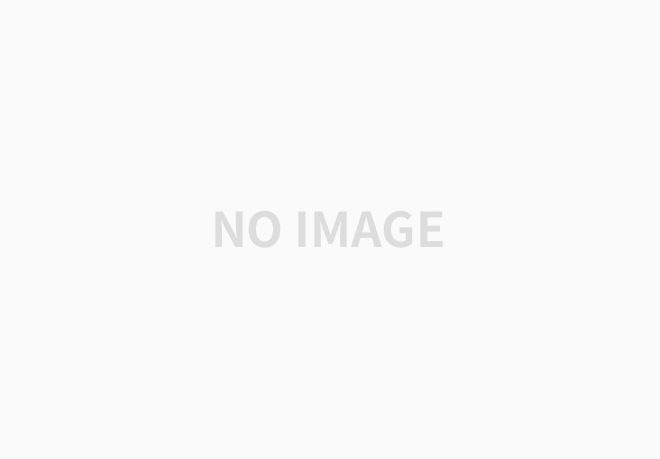
- Train Loss
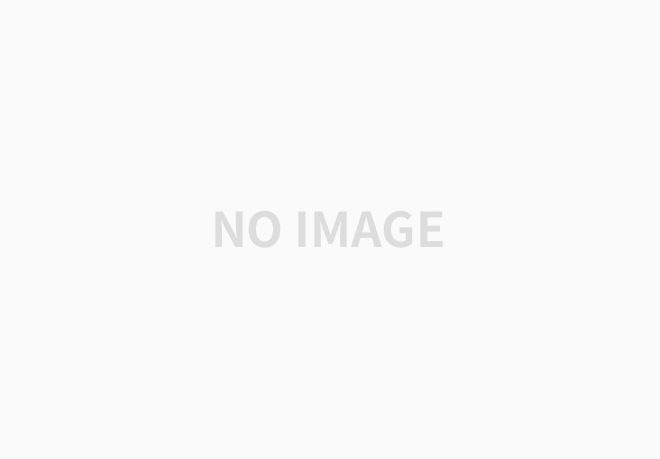
- Val Loss
- 약 25epoch이후 class loss, objectness loss는 상승하는 경향 -> overfitting?
- but, mAP는 꾸준히 상승
- LB에서도 25epochs 부근 model성능이 가장 좋을까?
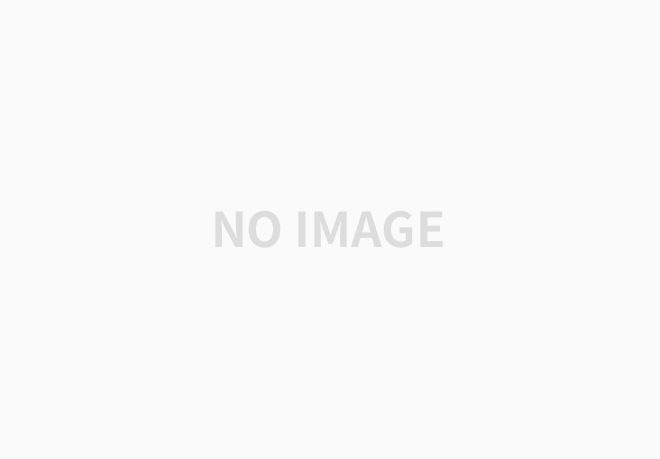
- PR curve
- mAP 상위 classes : Battery - 0.709, Plastic bag - 0.671, Glass - 0.684
- mAP 하위 classes : General trash - 0.246, Metal - 0.309, Plastic - 0.340
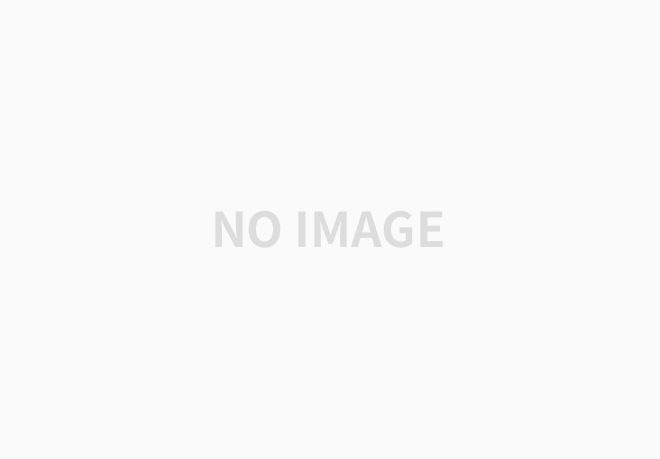
- Ground truth vs Prediction 비교
- large object에 대해서는 비교적 정확히 detection 수행
- bbox regression도 비교적 정확함
- classification error 다소 존재 (general trash <-> paper, glass <-> plastic)
- general trash mAP가 극히 낮은 이유?
- 기본적으로 Precision, Recall이 낮음
- Precision 관련 - '3194.jpg' 레이블링이 되어 있지 않지만 모델은 true라고 예측
- Recall 관련 - '3256.jpg' 레이블링이 되어 있지만 모델은 false로 예측
- 실제로 있는데 없다고 예측하는 경우, 실제로 없는데 있다고 예측하는 경우가 다수
- labeling기준이 모호함
- general trash에 포함되는 trash가 너무많아 컴퓨터 관점에서 feature가 너무 다양함
- 비교적 small object인 경우가 많음
- 기본적으로 Precision, Recall이 낮음
- 기타 실험 예정
- ensemble - 2 stage detector, 1 stage detector의 ensemble효과(?)
- yolov5 augmentation, 파라미터 튜닝
- general trash에서 small object 전처리 threshold를 좀 더 높인다면? 개선이 될지...?
- EfficientDet-b7은 왜 mAP가 낮은가... (LB : 0.302)